Typical machine learning methods used to analyze experimental imaging data rely on tens or hundreds of thousands of training images. But Daniël Pelt and James Sethian of Berkeley Lab’s Center for Advanced Mathematics for Energy Research Applications (CAMERA) have developed what they call a “Mixed-Scale Dense Convolution Neural Network” (MS-D) that “learns” much more quickly from a remarkably small training set.
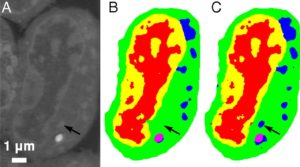
One promising application of MS-D is in understanding the internal structure and morphology of biological cells to identify, for example, differences between healthy and diseased cells. In one such project in Carolyn Larabell’s lab, the method needed data from just seven cells to determine the cell structure.
“This new approach has the potential to radically transform our ability to understand disease, and is a key tool in our new Chan-Zuckerberg-sponsored project to establish a Human Cell Atlas, a global collaboration to map and characterize all cells in a healthy human body,” said Larabell, a faculty scientist in the Molecular Biophysics and Integrated Bioimaging (MBIB) Division, Director of the National Center for X-ray Tomography (NCXT) located at the Advanced Light Source (ALS), and a professor at the University of California San Francisco (UCSF) School of Medicine.
Details of the algorithm were published in the Proceedings of the National Academy of Sciences (PNAS) and are accessible to the research community via the SlideCAM (Segmenting Labeled Image Data Engine) web portal, part of the CAMERA suite of tools for DOE experimental facilities.
Read more in the Berkeley Lab News Center.