In a world striving for sustainability, understanding roots—the dynamic interface between plant and soil—is crucial. To that end, Berkeley Lab scientists from the Applied Mathematics and Computational Research (AMCR) and Environmental Genomics and Systems Biology (EGSB) Divisions developed RhizoNet, which harnesses the power of artificial intelligence (AI) to automate the process of root image analysis with exceptional accuracy. This pioneering tool, detailed in a study published in Scientific Reports, transforms the study of plant roots, offering new insights into root behavior under various environmental conditions.
EGSB recently introduced the latest version (2.0) of EcoFAB, a novel hydroponic device that facilitates in-situ plant imaging by offering a detailed view of plant root systems. Developed in collaboration with the DOE Joint Genome Institute and the Climate & Ecosystem Sciences Division, EcoFAB is part of an automated experimental system designed to perform fabricated ecosystem experiments that enhance data reproducibility. EcoBOT, the image acquisition system for EcoFABs, offers research teams the potential for systemic experimental monitoring—as long as data is analyzed promptly
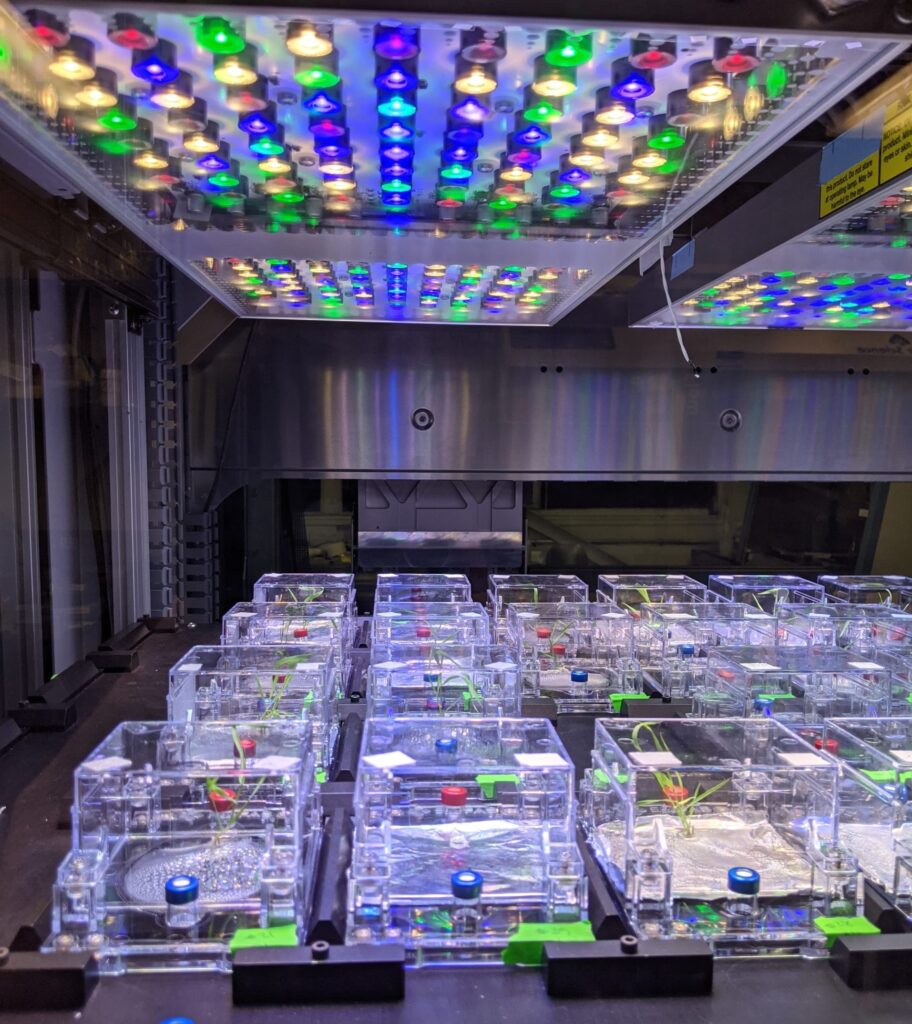
RhizoNet processes color scans of plants grown in EcoFAB that are subjected to specific nutritional treatments, addressing the scientific challenges of plant root analysis. Using an advanced deep learning-based backbone based on a convolutional neural network, this new computational tool semantically segments plant roots for comprehensive biomass and growth assessment, changing the way laboratories can analyze plant roots and propelling efforts toward self-driving labs. To illustrate this, the Scientific Reports paper details how the researchers used EcoFAB and RhizoNet to process root scans of Brachypodium distachyon (a small grass species) plants subjected to different nutrient deprivation conditions over approximately five weeks. These images, taken every three to seven days, provide vital data that help scientists understand how roots adapt to varying environments.
“We’ve made a lot of progress in reducing the manual work involved in plant cultivation experiments with the EcoBOT, and now RhizoNet is reducing the manual work involved in analyzing the data generated,” noted Peter Andeer, a research scientist in EGSB and a lead developer of EcoBOT, who collaborated with AMCR’s Daniela Ushizima on this work. “This increases our throughput and moves us toward the goal of self-driving labs.”
Resources at the National Energy Research Scientific Computing Center (NERSC)—a U.S. Department of Energy (DOE) user facility located at Berkeley Lab—were used to train RhizoNet and perform inference, bringing this capability of computer vision to the EcoBOT.
EGSB’s Trent Northen and and AMCR’s James Sethian also contributed to this work. This multidisciplinary group of scientists is part of Twin Ecosystems, a DOE Office of Science Genomic Science Program project that integrates computer vision software and autonomous experimental design software developed at Berkeley Lab (gpCAM) with an automated experimental system (EcoFAB and EcoBOT) to perform fabricated ecosystem experiments and enhance data reproducibility. The work of analyzing plant roots under different kinds of nutrition and environmental conditions is also part of the DOE’s Carbon Negative Earthshot initiative RESTOR-C—The Center for Restoration of Soil Carbon by Precision Biological Strategies.