Traumatic brain injury (TBI) affects approximately 4 million people in the United States annually and costs the world economy $400 billion a year. Current classification frameworks group TBI patients into just three categories—mild, moderate, or severe—based on their clinical presentation. A critical first step toward personalized treatments for TBI (and other complex medical conditions) is to increase the precision with which patient outcomes can be predicted. This involves the challenging task of gleaning and leveraging clinically relevant understanding from large biomedical datasets.
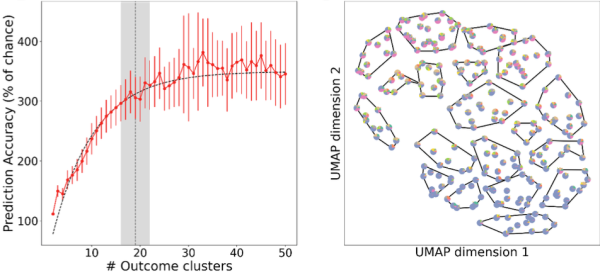
Using an extensive dataset created by the Transforming Research and Clinical Knowledge in Traumatic Brain Injury (TRACK-TBI) collaboration, and machine learning capabilities developed by data scientists in Berkeley Lab’s Computing Sciences Area, a team of researchers achieved a greater than six-fold improvement in precision over current TBI classification. After analyzing hundreds of simulations on the Cori supercomputer at the National Energy Research Scientific Computing Center (NERSC), they identified 19 types of TBI patient outcomes that can be predicted from intake data.
“Our goal with this collaborative project was to bring together clinical experts in TBI who have complex, high-dimensional datasets with data scientists who have expertise in data-driven discovery,” said Kris Bouchard, who leads the Computational Biosciences Group in the Computing Sciences Area and is a staff scientist in Biosciences’ Biological Systems and Engineering (BSE) Division. “We were able to develop and apply new machine learning methods to address outstanding challenges found broadly across the entire medical community, not just TBI.”
The study, which involved researchers from Berkeley Lab, UC San Francisco, the Medical College of Wisconsin, UC Berkeley, and TRACK-TBI, was published in Nature Scientific Reports.