When is AI alone adequate for answering scientific questions? It depends.
Artificial Intelligence (AI) took the structural biology world by storm when Google parent company Alphabet’s DeepMind AlphaFold software was released in 2021. It was a major breakthrough in three-dimensional protein structure prediction, promising highly accurate models for proteins of unknown conformation. With this advance, some in the field assumed that there would no longer be a need for scientific experiments to determine protein structure.
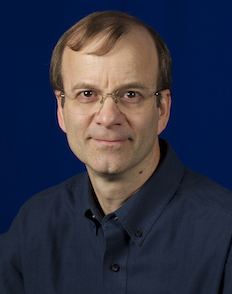
However, a multi-institutional team led by Tom Terwilliger (pictured, right) from the New Mexico Consortium and including researchers from Berkeley Lab have shown that—as with other similar tools that promise to make quicker work of solving structures—there is still a need for empirical evidence and human contributions. In a study published in Nature Methods, the team performed a rigorous analysis of AlphaFold predictions, comparing them with both high-quality experimental data and experimentally determined structures. Their results led them to conclude that AI-based protein structure predictions are best considered to be exceptionally useful hypotheses, and that experimental measurements remain essential for confirmation of the details of protein structures.
The international team includes developers of the Phenix software suite from the New Mexico Consortium, Los Alamos National Laboratory, Duke University, Cambridge University, and Berkeley Lab. Phenix is used by structural biologists around the world to solve macromolecular structures from X-ray, neutron, and cryogenic electron microscopy (cryo-EM) data. The researchers combined their detailed knowledge of the chemical and physical properties of protein structure with experience building models from experimental data to probe deeper into the accuracy of AI-generated models and how they are best used. They devised several methods to compare the AlphaFold models with the experimental information and models available in the Protein Data Bank (PDB).
“We found that while AlphaFold predictions are often astonishingly accurate, many parts do not agree with experimental data from corresponding crystal structures,” said Terwilliger, corresponding author of the study. X-ray crystallography is a common technique used to gather electron density data from proteins arrayed in crystals and determine the proteins’ molecular and atomic structure. In particular, the team found that AlphaFold predictions are less accurate at representing the contents of a crystal than the models of proteins obtained from experimental data deposited in the PDB.
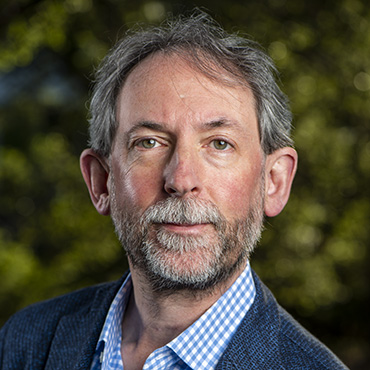
“We know from our experience that experimental determinations of protein structures contain errors, so we wanted to see how the errors in the AlphaFold predictions compare,” said Paul Adams, Associate Laboratory Director for Biosciences at Berkeley Lab and Phenix Program Director (pictured, left). AlphaFold predictions come with a confidence score that indicates how likely the model is to be accurate. Therefore, the team used a clever method to show that even the highest-confidence AlphaFold predictions have errors that are about twice as large as those present in experimentally determined structures. What’s more, Terwilliger noted that not all protein structures are predicted with very high confidence, and lower-confidence predictions are much less accurate than experimentally determined ones.
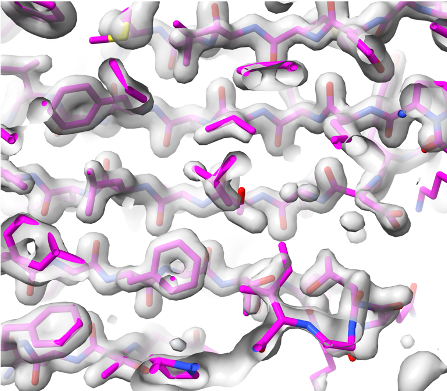
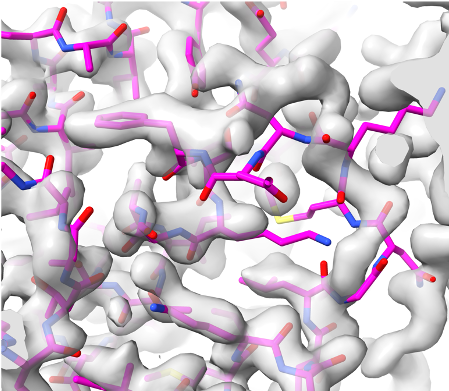
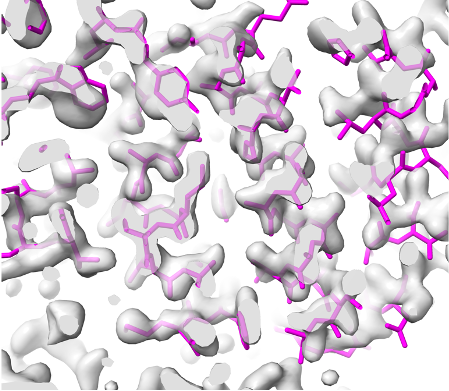
Overlaid on experimental electron density, left to right: a near-perfect AlphaFold prediction, an incorrect one, and a distorted one, all predicted with very high confidence. (Credit: T. Terwilliger, The New Mexico Consortium)
The team found that AlphaFold predictions with the highest level of confidence have approximately twice the errors of high-quality experimental structures. “And about 10% of these highest-confidence predictions have very substantial errors,” Terwilliger said, “which makes those parts of the predicted models unusable for detailed analyses such as those needed for drug discovery.”
The researchers also note that AlphaFold prediction does not take into account the presence of ligands––which are molecules that affect the protein’s structure or function when bound––as well as ions, covalent modifications, or environmental conditions. Therefore, it cannot be expected to correctly represent the many details of protein structures that depend on these factors.
Adams said that the level of accuracy needed in the AI prediction is tied directly to what scientific questions are being asked. “If a scientist is studying the evolution of proteins by making global comparisons, the AlphaFold models are very useful,” he said. “However, if a researcher wants to study ligand docking for structure-based drug design, there is no substitute for experimental data that provides a higher confidence in amino acid side chain conformation.”
Even as AI gains traction, we will always need some human input to analyze results and apply AI-generated hypotheses to the greatest effect.
The team’s results highlight the importance of employing AI models of proteins, like those predicted by AlphaFold, as exceptionally useful hypotheses that should be confirmed with experimental structure determination. “While these AI models have the power to revolutionize the field, we have been careful to note what kinds of structure and environmental elements are not taken into account,” said Terwilliger. “We also provide multiple test cases to underscore the uncertainty of predictions, like when the same amino acid sequence can generate more than one crystal structure.” This is particularly necessary, he noted, when looking at functional areas in a protein.
“Our analysis clearly shows that AlphaFold models can be very good, but that they are not always good, even if they are predicted with high confidence,” said Adams. The team’s results demonstrate that experimental structure determination remains a crucial part of structural biology, and biology in general. “Even as AI gains traction, we will always need some human input to analyze results and apply AI-generated hypotheses to the greatest effect.”