The exponential growth of biomedical and clinical data sets over the past decade presents an unprecedented opportunity to better understand human health and disease. However, the diverse, siloed, and non-standardized nature of these data is a barrier to unlocking their potential. Ideally, these data would be mined collectively to provide insights into the relationship between molecular and cellular processes and the signs and symptoms of diseases.
The Biomedical Data Translator (Translator) program was launched in 2016 with funding from the National Center for Advancing Translational Sciences (NCATS) within the National Institutes of Health. In fiscal year 2020, NCATS awarded approximately $13.5 million to establish the initial Biomedical Data Translator Consortium, comprising teams of scientists, physicians, bioinformaticists, and programmers. The consortium is tasked with collaboratively developing a knowledge graph–based platform—the Translator system—for combining, searching, and reasoning over biomedical data to derive knowledge and accelerate clinical discovery.
In a pair of recently published papers, consortium members detailed new features, functionality, and applications of the Translator system and its underlying data model, the Biolink Model.
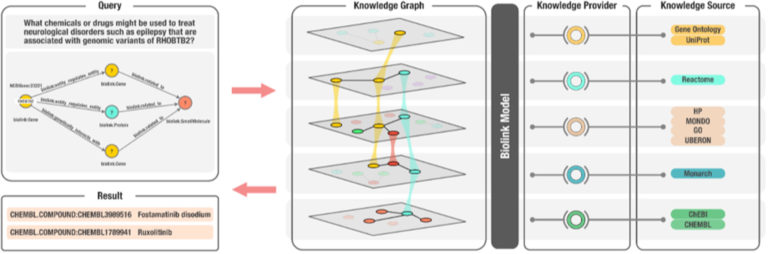
Development of the universal, open-source Biolink data model was led by researchers in the Environmental Genomics and Systems Biology (EGSB) Division at Berkeley Lab. The model is intended to standardize ontologies, naming conventions for nodes/entities in knowledge graphs, and the relationships between entities. Additionally, it maps comparable elements between ontologies, allowing search and comparison across disparate data sets.
“It is inspiring to see experts from a wide variety of domains communicate and collaborate on a shared model,” said Sierra Moxon, a software developer in EGSB. “Biolink Model establishes a common language to communicate with, and that’s the first step to solving hard problems together.”
“One of the main needs of Translator was a common dialect for organizing, representing, and exchanging knowledge between knowledge providers, subject matter experts, and machines,” said Deepak Unni, an affiliate software developer in EGSB. “Biolink Model addresses this need by providing a harmonized data model that tackles challenges with knowledge representation and provides a foundation upon which intelligent applications can be built.”
Additional EGSB staff who contributed to the papers include: data scientists Harry Caufield and Marcin Joachimiak, program manager Nomi Harris, and staff scientist Chris Mungall.
Read more from the University of North Carolina at Chapel Hill’s Renaissance Computing Institute (RENCI).